- Feature
- Treating data as assets
Treating data as assets
By Thomas C. Redman
About a dozen years ago, the expression “manage data assets” entered the business lexicon.
Data can be built into products and services, using more and better data improves business decisions, and data is the input for game-changing opportunities such as artificial intelligence. Thanks to social media and the Internet of Things, the sheer volume and variety of available data is growing by leaps and bounds.
Still, most people find the concept 'manage data assets' slippery. It sounds like a good idea (and it is!), but what does it really mean? And what must companies and managers do differently? My goal in this article is to provide a simple answer to the first question and a partial answer to the second, focusing on the steps that companies and all managers can, and should, take in the next few months.
To answer the first question, it is useful to study how companies manage things they have traditionally viewed as assets and to transfer those insights to data. Companies manage physical assets, financial instruments, and (to a lesser degree) people, as assets. And one observes the following:
- Companies take care of these things: They maintain plant and equipment, they lock up the petty cash, and they invest in their people.
- They put these things to work: They use their physical assets and employ their people to make products they can sell at profit. Public sector organizations put their assets to work to advance their missions.
- They advance management systems best suited to those things. This area is a bit more involved. For now, I will simply note that physical plant and people are different sorts of assets and companies adopt different styles of management accordingly.
I propose that something qualifies as an asset if those responsible meet three criteria:
- They take care of it,
- They put it to work, and
- They manage it appropriately.
This approach is simple and powerful. These criteria apply to companies, government agencies, non-profits, and people.
It is easy enough to adapt these criteria to data. Readers may also wish to evaluate whether their organizations meet them. For data, taking care is largely about quality and security. Specifically, companies must invest to:
a. Ensure they have the data needed to conduct operations, manage the business, and plan their futures. In particular, they must make sure these data are “fit for use.”
b. Keep their data secure from the prying eyes of others.
It appears to me that many companies do a reasonable job securing their data. Quality is another matter - most data simply do not meet basic quality standards. This poor quality is extremely costly: An IBM estimate puts the tab at USD 3.1 trillion /per year in the United States. A typical company’s share is an astonishing 15 to 25 percent of revenue.
There are many ways to put data to work. The spirit of this criterion is that a company has explicitly thought through its options, developed a plan, and is working that plan. But most companies readily admit they do not derive a fraction of the value their data offer.
Finally, data have many properties unlike other assets. Perhaps the most tantalizing is that data can be copied and shared at very low cost - a virtually unlimited number of people can use the same data for many and varied purposes. You simply cannot share a physical asset, dollar, or an employee in the same way. This property illustrates data’s enormous potential! But organizational silos and technical issues get in the way and most data are not shared. This exemplifies how most companies fail the manage data appropriately criterion.
Today, most companies fail all three criteria - after all, these criteria are deliberately tough and the notion that data are assets is relatively new. So, companies and all managers must take near-term steps that will both help them gain experience and demonstrate the enormous benefits that managing data professionally and pro-actively bring.
For companies, the first step is to get their data teams out of their information technology departments. There is a natural tendency to reason, “data are in the computer, therefore they must be tech’s responsibility.” By this logic, since people work in buildings, they should be managed by Facilities Management. Data and information technologies are different sorts of assets that require different management systems. When managed by IT, data are given second-class treatment, exactly counter to their desired status as assets. More than any single factor, improperly assigned responsibilities hold companies back when it comes to treating data as an asset.
If data are assets on par with capital and people, it stands to reason that the “Top Data Job” will be on the same level as the Chief Financial Officer and Head of Human Resources. This won’t happen for some time, so for now companies should take the first step by finding a better spot for their data teams.
In the rest of this article, I turn my attention to individual managers. They have outsize roles to play, though the vast majority do not give data much thought. This is unfortunate because one of the fascinating properties of data is that they are also 'meta-assets', informing the maintenance of physical equipment, the deployment of capital, and programs to increase employee satisfaction. No one can do his or her job without data and for this reason alone, people, at every level, in every department are well-advised to treat data as assets.
Where to begin? I recommend three first steps, all of which can be completed in four to six months. First, pick a small set of data for your initial focus. Managers complain about a veritable tsunami of data, coming at them from all over. But the practical reality is that most data is never used for anything. Only a small fraction are “absolutely essential,” while some qualify as “pretty important,” and more as “nice to have.”
To narrow your focus, pick a small number of your most important tasks and then consider the data you need to do that work. For example, if your job involves maintaining solar arrays, consider the data needed to do that job well.
The next step is to baseline the quality of the selected data. One good way to do so is using the Friday Afternoon Measurement (FAM), so called because it is simple enough to conduct on a Friday afternoon. To do so, assemble 10-15 critical data attributes for the most recent 100 instances of the data selected above - essentially 100 data records. To maintain solar arrays, such attributes may include: PV module type, nominal power, peak power according to the flash test, and module temperature. Then, with a small team, work through each record, marking obvious errors. Lastly, count up the total of error-free records. This number, which can range from 0 to 100, represents the percent of completely correct data, the data quality (DQ) Score.
Most managers, quite naturally, expect their data to be pretty good and FAM provides a real shocker! In the most comprehensive study of actual data quality levels, the average score was DQ = 53% and many scores were lower. Only 3% met basic quality standards. A wake-up call if there ever was one!
The third step is to make an improvement. FAM also provides error rates for each attribute. One usually finds that two or three attributes account for 80% of the errors (the Pareto principle in action). Pick one of those attributes, find out where that data is created, find the root cause, and eliminate it.
Plenty of managers have taken these steps and made big improvements. In so doing, they have begun to improve their team’s performance and position themselves for far brighter futures! Even better, these simple exercises (narrowing the focus, FAM, and improvement) provide a tantalizing glimpse of the enormous benefits that are there for those who implement them across entire departments, business units and the enterprise.
About the author
Thomas C. Redman Ph.D. “the Data Doc,” President of Data Quality Solutions, helps start-ups and multinationals; senior executives, Chief Data Officers, and leaders buried deep in their organizations, chart their courses to data-driven futures, with special emphasis on quality and analytics. Tom’s most important article is “Data’s Credibility Problem” (Harvard Business Review, December 2013) He has a Ph.D. in Statistics and two patents.
Text ©2018 Thomas C. Redman, Ph.D. Used with permission.
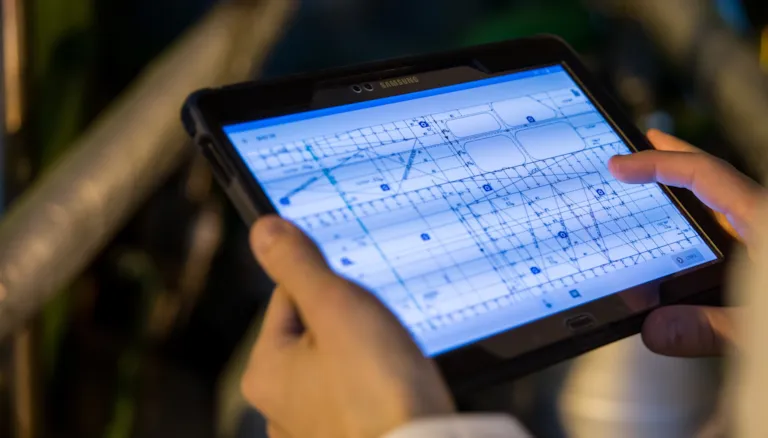
The road to data quality
Q&A with DNV data management specialists, Per Myrseth and Jarl S. Magnusson
DNV has always been entrusted with data by its customers, and the quality of data insofar as it relates to mission- and safety-critical systems and processes has always been a key concern. Here, two of our leading data management specialists, Per Myrseth and Jarl Magnusson explain what DNV is doing to tackle the data quality challenge.
Are our customers concerned about data quality?
Perhaps not as much as they should be, at executive management level – and the alarming statistic quoted by Thomas C. Redman above about the cost of bad data bears testament to that.
But … what we are seeing is that top management at our customers’ firms are becoming increasingly aware of the value of data, and of the central role it will play in enabling more competitive operations in the future. There is a great willingness to invest in use cases to prove this – for example through machine learning, predictive maintenance and real-time decision making. But all too often the experiment is stopped or de-scoped owing to data quality issues. This can be disappointing, but we’ve also seen cases where management has been pleased to uncover underlying data problems – which of course is the right attitude, from where we sit!
Can you give some typical examples of data quality failures?
Sure. It all depends on what you wish to use the data for. We prefer to think in terms of whether data is ‘fit for use’. In some cases you might require great precision, and others not.
Usually the problem is associated with transforming data into information. To do that you have to have data about the data – or as Redman says, ‘metadata’. For example, the number 8 is data, but what does it mean by itself? 8 cm starts to get you somewhere. But knowing what 8 cm relates to and when this was measured might be better still. If you wish to compare vibration and temperature data sets, but the data is not equivalently time stamped (with respect to time zone, seasonality, calibrated clock and so forth), the comparison can be worse than worthless. Indeed, faulty information about data can be fatal. If an airliner has frozen airspeed sensors sending incorrect air speed readings to the pilot, the consequences can be catastrophic, as we know all too well from history.
So, going back to our statement about ‘fit for use’: Traditionally, data has been designed and created for usage in silos for silo-based decisions. But when you’re wanting to make complicated decisions combining many disparate data sets, the need for data quality – for example, precision, completeness and reusability– rises exponentially.
What does DNV know about data quality?
DNV has sometimes been referred to as a data refinery. To perform our work – to make rules and standards, or to verify performance against regulations – we have always taken on board enormous amounts of data, and the volume of data is growing rapidly. Of course, we’ve naturally been concerned about the quality of the data itself, and so data quality has been hardwired into our modus operandi. This concern goes right back to our roots in the 19th century, and forward-thinking colleagues like Friedrich Schüler who was the first to use statistical evaluation of big data sets from actual ships to develop rules.
In recent years we have extended our concern about the quality of the data we use, to the quality of the data our customers are using for decision making. DNV has participated in creating the standard ISO 8000 on data quality, and have developed a Recommended Practice on a framework for data quality assessment. We have issued a position paper on sensor reliability, and we are currently busy with a recommended practice covering the quality of algorithms.
How do you assess the quality of algorithms?
One way of doing this is to ensure the optimal quality of the data that a machine learning algorithm, for example, may be using as a 'training set'. If a machine is learning using bad data, then the new algorithms are going to be bad. The ultimate prize will be for machines to recognize data quality problems and either isolate those data or develop routines to compensate accordingly.
How do you fix bad data?
Data cleansing is invariably expensive and time consuming. It is far more preferable to fix data problems at the root. We find that eliminating a single root cause can prevent thousands of future errors. So, you need to go from fixing bad data to fixing bad data management. For that, we recommend a systematic approach.
The steps towards a robust data quality framework are straightforward, but may involve some retooling of technology and adjustments to processes.
Start by deciding what you need the information for: is it to maintain or enhance operations, better use personnel, predictive maintenance, improve safety, reduce environmental impact, and so on. Once you have a handle on your information requirements, that informs your data requirements – e.g. you may need high precision data, which creates specific infrastructure needs; or you may need more high-level aggregate data, which creates another set of requirements.
The next step is a maturity assessment of your organisation: how knowledgeable are your people about data quality tools, procedures and standards? Couple that with formally checking the quality of existing data, against the rules and requirements you wish to apply. This is, if you like, a more formal application of the ‘Friday Afternoon Management’ sense-check that Redman writes about above. You can try our Data management maturity self-assessment tool to identify gaps and set some initial goals.
The maturity and data quality assessments are inputs to a risk assessment, and help an organization to adopt a risk-based approach to improving data quality. If you know where the quality issues are you can compensate for that – rather than making decisions on the basis of bad data. You can also embark on a process of continuous improvement, improving where the data is born via a sensor system assessment, and how it is processed, via an algorithm assessment.
What's next for data quality?
The risk-based approach we’ve just described hasn’t gone mainstream, but is an obvious path for organizations wishing to improve their data quality.
And there are compelling reasons to do so in this ever more connected world. As Redman states, “…a virtually unlimited number of people can use the same data for many and varied purposes.” That is the premise behind platform collaboration, and indeed DNVs Veracity data platform. But networked innovation and co-creation is undone if the data are bad and carry no power for advanced analytics.
That is why, in our view, the organizations that today are sweating the hard yards of improving their data quality frameworks will be the ones with competitive advantage in a tomorrow’s data driven world. In fact, we would go as far as saying that quality-marked data (the digital equivalent of the 'Woolmark') will trade at a distinct premium in the future.
Further reading
Read offline
Download this article as a PDF
Data quality self-assessment
Are your data quality procedures good enough?
Instant visualization of data quality
Data quality assessment is a method to verify that data meet the expectations of users or systems utilizing the data.
Organizational Maturity Assessment
Assess your organization’s capability to capture, measure, use and share data.