DNV Group Research & Development collaborates with nine universities across the world. This includes the sponsoring of professorships and PhD students, lectures by our employees and the supervision of students. One of the partnerships is with the Fudan University’s School of Data Science in Shanghai.
University relations
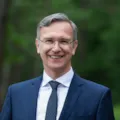
For us at DNV, using AI for delivering our services in a more advanced way is relatively straight forward. But as certification service provider, we have the assurance of AI algorithms and AI-enabled systems in our research focus. Clearly, one day we want to certify AI. Therefore, we have established this collaboration and we are working with Professor Yanwei Fu at the School of Data Science of Fudan University, one of the most prestigious universities in China.
- Group Research and Development Director ,
- DNV
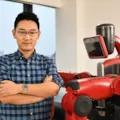
AI is a very comprehensive topic and how to assure AI is a huge task. During our collaboration we have already made some progress. For example, we have proposed a bow-tie model approach which is based on concepts already in use in industrial applications by those that use the risk management model.
- ,
- School of Data Science of Fudan University, China
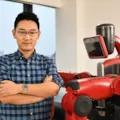
I believe our interdisciplinary research work and expertise provides a very good basis for collaboration with DNV, which is a recognized global company with a strong focus on helping its customers achieve safe and sustainable operations. Through our collaboration I think we can push AI assurance to a higher level and make a real difference to industries and societies,” he concludes.
- ,
- School of Data Science of Fudan University, China