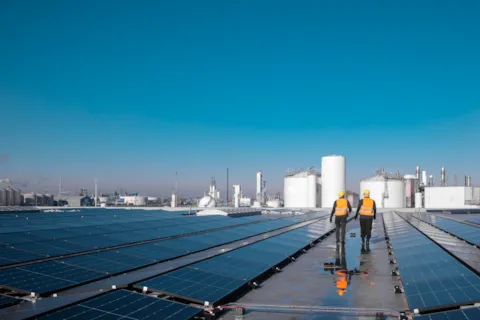
From silos to systems
This special report explores why whole energy systems thinking is critical to accelerating the energy transition.
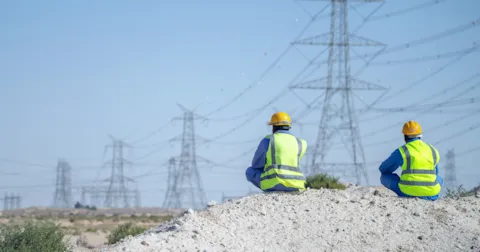
Short-term volatility, long-term optimism
More than 1,100 senior energy professionals shared their views on how economic and political uncertainty is reshaping the industry’s outlook and priorities.
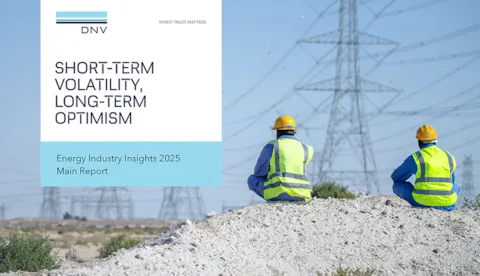
Main report: Short-term volatility, long-term optimism
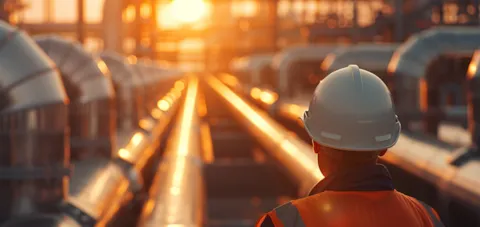
Pipeline Insights 2024: The story of change and constancy
The Pipeline Insights 2024 report offers an unparalleled view into the heart of the pipeline industry. Drawing from the responses of 142 pipeline professionals across 40+ countries, this comprehensive study provides a nuanced understanding of the sector's confidence levels, investment priorities, and strategic outlook.
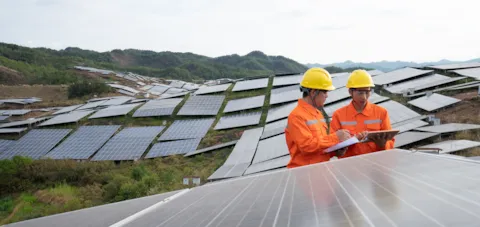
Leading a data-driven transition
This special report explores how excellence in digitalization is helping energy industry organizations grow greener, faster, and better.
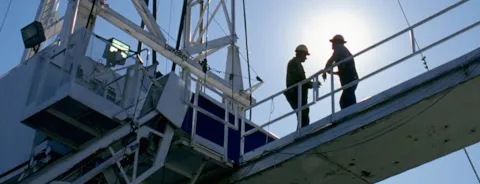
THE PARADOX OF PETROLEUM
By gathering insights from nearly 450 senior oil and gas professionals, this report explores the dilemmas of the sector, in addition to the trends and near-term outlook.