PHMSA’s Gas Mega Rule: CFR 192.917
PHMSA’s Gas Mega Rule amendments to 49 CFR 192 demand urgent attention from gas transmission pipeline operators, requiring significant adjustments to integrity management programs for continued regulatory compliance. With limited resources, it's crucial that every dollar spent improves pipeline safety and reliability performance effectively.
Watch this 30-minute ‘Ask SME a Question’ session as DNV’s Subject Matter Expert, Alex Woll, unpacks the key changes to Subpart O, focusing on their impact on Risk and PMM requirements for your IMP. He provides clarity and expert guidance and answer specific RIN2 implementation questions for the audience.
Read the Q&A from the webinar:
Answer: Demonstrating compliance with risk reduction activity requirements involves a two-fold approach: establishing a decision-making framework and documenting your Preventive and Mitigative Measures (PMMs). First, you need to have a clear decision-making framework. This framework is typically included in your Integrity Management Program (IMP). It's important to note that PHMSA doesn't prescribe a specific framework, so as a company, you'll need to decide on an approach that works for your organization. Many operators in the US opt for a corporate risk threshold or a corporate risk matrix that defines different thresholds within it. This decision-making framework serves several crucial purposes. It helps you determine when action needs to be taken based on your risk results. It should clearly outline at what risk levels you need to intervene because the risk has exceeded your company's risk appetite. Additionally, the framework should guide you in determining when you've done enough. If you're implementing PMMs and they're having a certain effect on your risk, your framework should help you decide when you've sufficiently mitigated the risk. The second component is documenting your consideration and selection of PMMs. There are various prescriptive PMMs that you're required to consider. To document this process effectively, you should develop a recording mechanism. This could be as simple as an Excel sheet where you list all the PMMs for each asset. For each PMM, you should evaluate its impact on risk reduction and its cost to implement. This is effectively a cost benefit analysis for each potential measure. |
Answer: Sensitivity analysis is a powerful tool for understanding and enhancing risk models, but it's important to approach it in a way that provides meaningful insights. There are various methods of conducting sensitivity analyses, each with its own strengths and limitations. Some operators use a simple mathematical approach that looks at the weightings associated with factors in their model. This provides a static sensitivity analysis and doesn't account for data quality and essentially only represents model uncertainty. As a result, its usefulness can be limited. On the more complex end of the spectrum, some operators employ full Monte Carlo sampling and then run statistics on how this sampling impacts risk results. This involves sampling your inputs and analyzing their effects on your outputs. While this can provide detailed insights, it often combines data quality and model uncertainty, making it difficult to distinguish between the two. This can lead to situations where a factor seems to have an outsized impact on the results, but you can't tell if this is due to data quality issues or the model structure itself. Given these limitations, a more effective approach might be to keep data uncertainty modeling separate from model uncertainty modeling. For data uncertainty, you could use a P50 (median) and P99 (worst credible case) scenario to model the data impact. Separately, for model uncertainty, you would filter for areas where you have good quality data and then compare your results to industry trends. Building on this foundation, a layered sensitivity analysis can provide a more comprehensive understanding of your risk drivers. This approach aligns well with the PHMSA requirements, which state that you need to consider the factors contributing to both likelihood and consequence. In a layered sensitivity analysis, you would start at the highest level by identifying which threats or consequences contribute most to a risk value. Then, for each of these threats and consequences, you would determine which factors contribute most significantly. You could continue this process down to the input level if needed. This layered approach offers several advantages. It provides a clear picture of what's driving your risk at different levels of detail. You can perform this analysis at various scales - for a specific line, for your entire system, or for any grouping in between. Each of these analyses would provide different insights:
By understanding your risk drivers at these different levels, you can more effectively select PMMs that are tailored to address your specific risk profile. This approach helps you not just in complying with regulations, but in truly enhancing the safety and efficiency of your operations. |
Answer: It's crucial to keep data uncertainty and model uncertainty separate to effectively address each issue. For data uncertainty, operators can employ a probabilistic approach. In the most advanced cases, this means treating all data inputs as probability distributions rather than single numeric values. This approach would require Monte Carlo sampling of these distributions, resulting in intermediary calculations, probabilities, consequences, and overall risk being expressed as probability distributions. However, this level of complexity may be unnecessary for most operators, as they are typically concerned with the most likely scenario and, in high-consequence locations, the worst feasible case. A simplified approach would be to identify two scenarios: the most likely case and the worst-case scenario. This can be done by asking users or Subject Matter Experts (SMEs) to provide these estimates where data is not explicitly known or by using industry defaults. The difference between the risk results of these two scenarios represents the uncertainty on the data side. For model uncertainty, the process involves gauging the conservatism of your model for each threat and consequence. Start by filtering your model results to areas where you have good key data quality indicators. You may need to conduct a sensitivity analysis to determine which data elements play the largest role in affecting your outputs. Once you have these filtered results, you can compare them to either your company's historical failure trends or the PHMSA 20-year or 10-year industry trends. To make this comparison, aggregate all your results where you have good key data quality indicators and normalize the number of failures per mile-year per threat. If you don't have enough company failures for a meaningful comparison (which is common for transmission operators), you can use the PHMSA statistics. Look at the 20-year trend, again normalizing per threat how many failures occur per mile-year. This comparison allows you to see whether each section of your model is over or under conservative when benchmarked against historical industry trends. You can then use this model uncertainty information to prioritize your model improvements. |
Answer: Prioritizing data cleanup is a critical task for improving the accuracy and reliability of your risk model. One effective approach is to establish a data hierarchy within your model. This hierarchy should be structured so that in the event of missing data for one of your inputs, you have multiple potential inputs ordered from best quality to least quality. By using the best quality data wherever it's available, you increase the likelihood of producing more accurate risk results. As you move down this hierarchy and encounter data in which you have less confidence or greater uncertainty, you should start modeling that data uncertainty as discussed earlier. The outputs of your data uncertainty modeling can then be used to drive your data improvement prioritization efforts. To facilitate this process, you should be reporting on your data confidence levels. By grouping these confidence levels, you can identify areas with the biggest discrepancies based on data uncertainty. Investigate what's driving these discrepancies - is there a common data set that's causing issues? If you focus on improving that particular data set, would it significantly decrease the gap between your worst-case scenario and most likely scenario? This approach helps you identify and address the "low-hanging fruit" to improve your data quality efficiently. Another strategy is to prioritize data cleanup on assets that have the highest risk in general or the highest consequence. These are areas where many operators are particularly sensitive, and improving data quality here can have a significant impact on overall risk management. If risk models are designed to use conservative assumptions where data is lacking, the higher risk assets will often lead to areas where data cleanup will be most crucial. Additionally, consider using sensitivity analysis to identify which inputs have the most significant impact on your risk results. Once you've identified these critical inputs, prioritize ensuring that these data sets are as complete and accurate as possible across as much of your asset base as you can manage. Remember, the goal is not to have perfect data everywhere - that's often impractical and unnecessary. Instead, focus on improving the data that has the biggest impact on your risk assessments and decision-making processes. |
Answer: This is a common concern among operators, but it's important to understand that having incomplete data doesn't preclude you from developing and benefiting from a risk model. In fact, the PHMSA risk model work group findings directly address this issue. Even in the executive summary, it's clearly stated that if you are concerned about your data quality, a probabilistic model is actually the best model to use. There are several reasons for this recommendation. First, a probabilistic model allows you to establish a data hierarchy. This means you can start with your best quality data and work your way down to less certain data sources. Where data is lacking, you can begin by prescribing your confidence in it. This approach allows you to use whatever data you do have effectively while also accounting for areas of uncertainty. Secondly, a probabilistic model provides a mechanism to visualize and quantify the impact of data uncertainty. This visibility is invaluable because it allows you to see where your biggest data gaps are and how they're affecting your risk assessments. You can use this information to prioritize your data improvement efforts, focusing on the areas where better data will have the most significant impact on your risk calculations. Moreover, using a probabilistic model when you have data quality concerns sets you up for long-term success. As you improve your data quality over time, your model becomes increasingly accurate and valuable. The structure of the model itself helps you identify where to focus your data collection and improvement efforts, creating a positive feedback loop that continually enhances the quality of your risk assessments. It's also worth noting that starting with a probabilistic model doesn't mean you need to implement the most complex version right away. You can begin with a simpler version that accounts for your current data limitations and gradually increase its complexity as your data quality improves. In essence, a probabilistic risk model, is not just for those with perfect data - it's a tool that can help you manage and improve your data quality over time while still providing valuable risk insights with the data you currently have. |
Answer: Determining the right balance in risk reduction efforts is a nuanced process that often involves applying the ALARP (As Low As Reasonably Practicable) principle. This principle is common in areas where regulators apply prescriptive risk criteria, such as in Canada, the UK, Netherlands, and Ireland. The ALARP principle essentially divides risk into three regions: unacceptable, broadly acceptable, and the region in between, often called the ALARP region. How you approach risk reduction depends on where your current risk level falls within these regions. If your risk level is close to or in the unacceptable region, cost-benefit analysis becomes less critical. In these cases, you may need to implement risk reduction measures even if the cost seems disproportionate to the benefit. For instance, you might be willing to spend $1,000 to reduce $1 of risk if it brings you below that unacceptable threshold. As you move closer to the broadly acceptable threshold, your approach should become more balanced. Here, you might aim for a one-to-one ratio, where you save a dollar in risk for every dollar spent on a Preventive and Mitigative Measure (PMM). To give this process more structure, you may want to develop a sliding scale within your risk management program that defines acceptable cost-benefit ratios at different risk levels. It's also important to consider factors beyond just the numbers. If you find yourself considering two PMMs that offer roughly equivalent risk reduction at similar costs, it's beneficial to involve your operations and maintenance teams in the discussion. These are the people who will be implementing the PMMs, and they often have valuable insights into the practicality and potential secondary benefits or drawbacks of different measures. Developing an overall strategy for your organization involves bringing together stakeholders from various departments. This includes people who are making day-to-day decisions about risk mitigation, financial officers who understand the company's resources, and executives who can speak to the company's risk tolerance. Through these discussions, you can develop a comprehensive understanding of how much your organization is willing to spend to avoid a dollar of risk at different risk levels. Remember that while you need to bring risks down to broadly acceptable levels to comply with regulations and ensure safety, there's a point of diminishing returns. Once you're within acceptable risk levels, you need to carefully consider whether further risk reduction is worth the additional cost and effort. |
Answer: PHMSA's requirement to consider multiple data sets related to cathodic protection (CP) might seem redundant at first glance, but there are good reasons for this approach. It's important to understand that the requirement is to integrate this pertinent information, not necessarily to use every in your calculations. The first thing to consider is data coverage. If you don't have complete coverage of one of these data sets across your entire system, you can use these seemingly redundant data sets to create a data hierarchy. This means that if you're lacking data in one location, you can supplement it with a slightly lower quality input from another data set. This approach allows you to maximize the use of available data and provide a more comprehensive picture of your CP system's performance. Even if you have complete coverage of one data set (let's say CP readings) across all your assets, integrating the other data sets can provide valuable additional insights. For example, if you see poor CP readings in an area, having CIS or CP system performance data readily available could help in diagnosing the issue or deciding on the most appropriate corrective action. Moreover, while you might not need all of this data for your current risk calculations, integrating it into your system sets you up for future model enhancements. As your risk model matures and becomes more complex, you may find ways to incorporate this additional data more meaningfully. For instance, consider CP system performance data. Most operators are primarily concerned with their current CP quality. However, if you start analyzing your impressed current system's amperage as a function of time and correlating that with good or bad readings, you can gain insights into your ground bed's performance over time. This could allow you to predict when you'll need to replace a ground bed, moving from reactive to predictive maintenance. It's also worth noting that having multiple data sets can serve as a form of data validation. If one data set shows anomalous readings, you can cross-check against the others to determine if there's a genuine issue or if it might be a data quality problem. In terms of practical implementation, you don't necessarily need to incorporate all of these data sets into your risk calculations immediately. A good approach would be to:
Periodically review how you're using these data sets and look for opportunities to incorporate them more meaningfully into your risk assessments. |
Answer: The question of Remote or Automatic Shut-off Valves (RMVs) is a complex one, and it's understandable that some operators might be skeptical about their cost-effectiveness. However, it's important to approach this issue with a comprehensive understanding of the regulations and the potential benefits of RMVs. Some operators argue that all the damage is done in the first 30 seconds following a rupture, and since RMVs aren't likely to actuate within that timeframe, they may not significantly reduce consequences. While this is a fair point, it may not fully align with PHMSA's intent and definition of a "rupture." To understand this better, we need to look at the context provided in the notes that accompanied the new valve rule. These notes offer insights into the initial intent of the rule, industry feedback, and where the final rule landed. One crucial aspect of the new valve rule was PHMSA's attempt to define the term 'rupture'. While it's true that in a guillotine rupture or full-bore rupture, most damage occurs in the first 30 seconds. However, the question arises: when does a leak become a rupture? PHMSA's definition of a potential rupture includes several scenarios, but a key prescriptive definition is a 10% pressure loss in a 15-minute timeframe. When developing an RMV approach and calibrating it to benchmark lines, we found that a failure size less than two inches on a 12-inch line could result in this level of pressure drop. This is considered a large leak, not necessarily a full-bore rupture. In such scenarios, depending on the flow conditions, you might have a situation where the pipe flow is not outpaced by the release rate, maintaining pressure. Even after isolation, this could result in a sustained vertical jet fire. The duration of this jet fire would be a function of your valve spacing, the pressure, and the diameter of the pipeline - essentially, the inventory at the time of isolation. This means that RMV valve spacing does indeed play a role in mitigating the consequences of such events. The valve rule was partly intended to inform what the valve spacing should be, particularly for new constructions. However, the inclusion of 192.935(c) in the regulations implies that RMV valve spacing should be considered in the RMV analysis for existing pipelines as well. In many cases, operators may find that the most cost-effective approach is not to reduce the severity of a failure by installing RMVs, but to reduce the likelihood of failure through other PMMs. By taking a comprehensive approach to risk management and clearly demonstrating how you're addressing the concerns that RMVs are meant to mitigate, you can make a strong case for compliance even without installing additional RMVs. However, it's crucial to keep in mind that this is a complex area of regulation, and interpretations may evolve. Stay engaged with industry discussions and be prepared to adapt your approach if regulatory expectations change. |
Meet our Risk Compliance Expert
Alex Woll, Pipeline Risk Team Lead, DNV
With years of hands-on experience as a risk and integrity engineer for major gas and liquid operators, Alex brings a wealth of practical knowledge to DNV’s Pipeline Risk Team. He has substantial experience implementing different risk model types and crafting different IMP approaches that seamlessly integrate risk and PMMs into broader integrity programs. Alex’s current focus is on driving risk modelling innovation to improve integrity decision-making – exactly where you need to be in this new regulatory environment.
Watch this webinar to clarify the impact of these regulatory changes on your pipeline safety practices and ensure a smooth implementation process for your organization.
Download the webinar material:
Explore the entire webinar series:
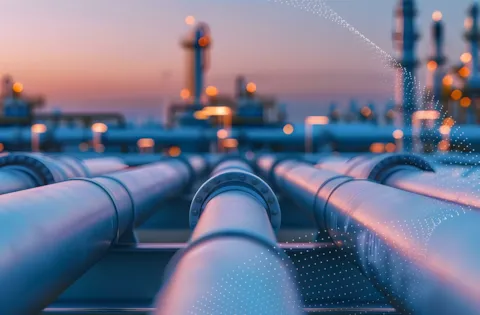
Ask SME a Question
Get expert guidance and answers to your questions about pipeline integrity and risk management from DNV’s top experts