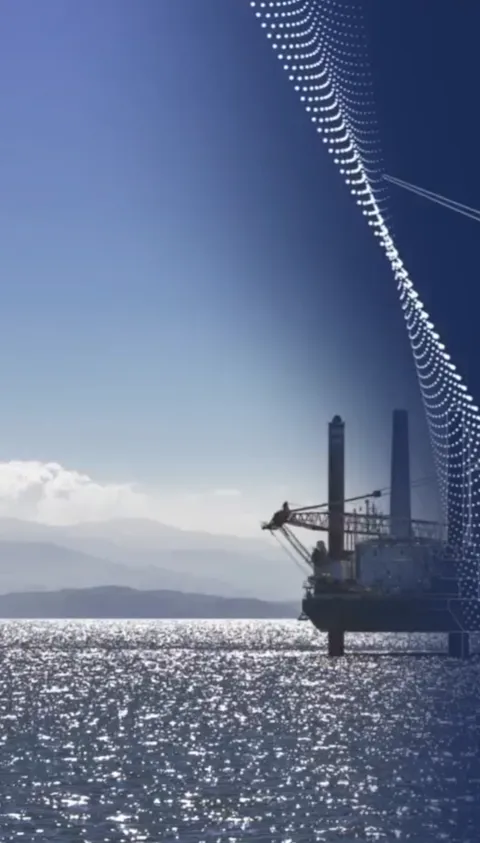
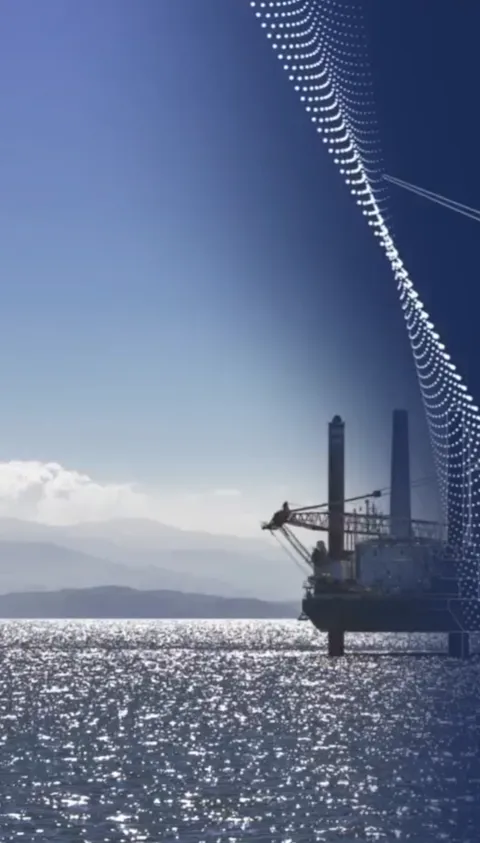
Digitalization is an important enabler of two key industry trends: decarbonization and decentralization, which are necessary to enable the energy transition.
An integral part of succeeding with digitalization is the realization that data is a valuable asset and should be treated as such. Data is the fuel powering digitalization, and high data quality is a prerequisite for getting return on your digital investments. Poor data quality now has a real financial impact, and companies cannot afford to ignore it. According to Gartner, the average financial impact of poor data on businesses is $15 million per year.1
Many of our customers report that they cannot trust their data. This is also confirmed by a Deloitte survey, where more than two-thirds of executives (including CEOs) report that they are not comfortable accessing or using data from their tools and resources. 2
On the brighter side, it looks like more and more companies are aware of data quality issues and the importance of solving them. According to DNV’s special report “Leading a data-driven transition”, 74% of respondents across the energy industry will prioritize improving data quality and availability in the coming year.3
A key step on your digitalization journey is to focus on improving data quality and implement robust data governance processes to make sure that you can trust the data for critical decision-making.
What is data management and what are common challenges?
Data management ensures that important data assets are formally managed throughout the enterprise and that data governance goals are achieved to realize efficiency gains and value creation. In addition to focus on data quality, data management also covers management of data storage, retention, life cycle, legal compliance and intellectual property rights, value chains, metadata, business vocabularies, information risks and information security.
Common data management challenges include:
- Not understanding the state of your data and how this affects your business
- Not knowing how to start managing data and prioritizing improvement actions
- Lack of an established data culture
1“Follow these 5 steps to effectively design a compelling data quality improvement business case” Gartner, 2018.
2Deloitte’s 2019 Becoming an Insight-Driven Organization survey
3DNV’s 2024 Energy Insights special report “Leading a data-driven transition”
Data quality and management services
We help you get a better understanding of how data can support you in achieving your business goals. Taking a holistic approach through the lenses of people, process and technology, our experts will help your organization to assess your data capabilities, define clear goals and provide strategic guidance on how to meet those goals.
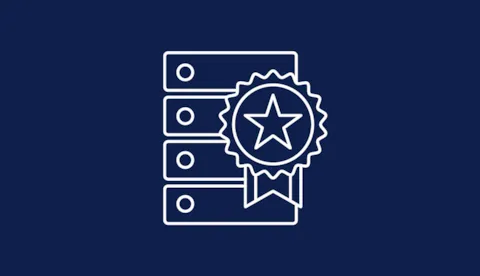
Data management capability assessment
Assess your organization’s data management ability with our comprehensive assessment to ensure that your data is fit for purpose. Take our free self-assessment.
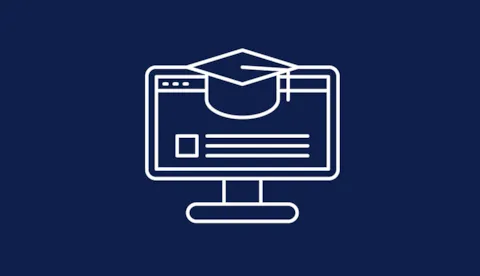
Data management course
Join this training course on data management to learn how to handle data, create value and avoid data quality issues.
Meet one of our data quality and management experts:
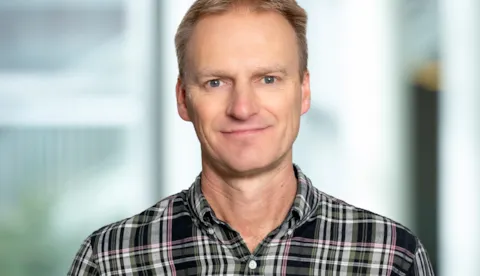
Karl John Pedersen, Principal Data Specialist
Customer success stories:
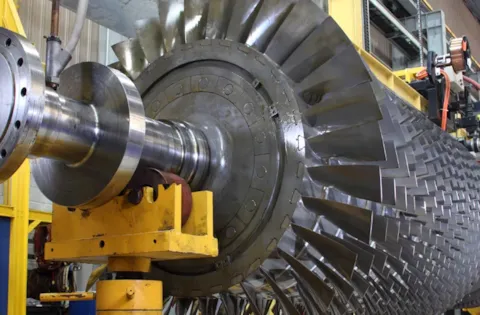
InterQ: Securing trust in data to enable “zero defect” manufacturing
DNV is bringing in expertise on assurance of data quality to the EUR 11 million project to enable safe and efficient manufacturing.
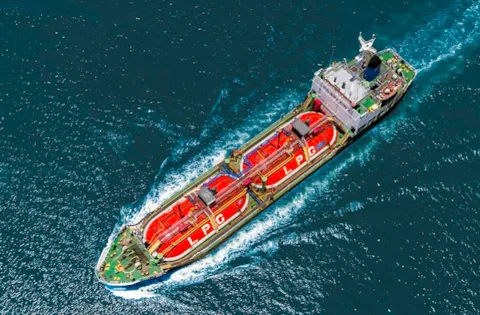
Bringing ESG data to life for Frontline
A key challenge with ESG performance tracking and reporting is the lack of high-quality data. To get a better understanding of Frontline’s current data management practices and data quality, DNV carried out a data maturity assessment where key improvement areas were identified.
Recommended practice for data quality assurance (DNV-RP-0497)
Download your complimentary copy
Data quality: The backbone of trustworthy AI
Read our frequently asked questions about data quality and management:
Data management is the practice of collecting, storing, organizing, and maintaining data to ensure its quality, accessibility, reliability, and security. Effective data management enables real-time monitoring, predictive maintenance, resource optimization, and better decision-making, ultimately enhancing overall performance and fostering innovation in the energy industry. |
Good quality data are fit-for-purpose and can be trusted, especially when used as a basis for critical decision-making. As the energy industry goes through a digital transformation where data are increasingly used in decision-making, data quality is of particular importance. Data quality is of particular importance as it underpins efficient operations, regulatory compliance, cost reduction, sustainability efforts, customer satisfaction, strategic planning, and risk management. |
Data governance is establishing the frame conditions for successful data management, including roles and responsibilities on the strategic, tactical and operational level. Data governance and management are enablers for creating business value and innovation by use of data. |
Considering the business value of good quality data enables an understanding of why data quality should be in focus. Achieving this initial understanding will help sell in the job that needs to be done to ensure that data is of good quality. |
Key use cases include scenarios where data are used to make decisions. An asset may be fully digital, where asset monitoring is carried out remotely using sensor data recorded locally at the asset. This data is used to make key decisions and must be trusted. The quality of the data is therefore paramount. The asset may be a remote windfarm, a subsea installation or an unmanned hydroelectric power station. |
Key benefits of data quality in the energy industry include:
|
Risks and challenges of poor data quality in the energy industry include:
|
Defining what good quality data, measuring the actual quality of data help in understanding what the quality of data is. The risk of using poor quality data can then be considered by domain experts. Proactive and reactive methods to fix data quality issues can be implemented. |
Data quality is a measure of the “health condition” of data, a measure of how well the data meets the requirements and expectations for its intended use within acceptable risk, cost and business needs. Data management provides the foundation to ensure good data quality and is the process of following the rules and procedures to ensure data is accurate, complete, consistent and up-to-date. Processes such as data validation and cleansing are part of the data management efforts to maintain high-quality data in the lifecycle. |
The regulatory landscape for data quality in energy industry varies with geographies and industry segments. The ISO 8000 data quality standard provides a generic approach to data quality which is applied to the energy industry. This can be applied using the best practices from Data quality management | Recommended Practice | DNV-RP-0497 |
Testing data quality starts with defining for a particular use-case what is meant by good data quality. This definition can be expressed as data quality requirements (e.g. that there are no blank records in data), which when achieved assures users that data are fit for purpose. Data quality can then be measure according to these requirements using for example simple checks in a database or using a data quality as a service. |
Data maturity shows how well data are managed. Well-managed data are accessible to users, have good data quality and in a data-driven organization contribute to good, trustworthy decisions. Assessing the current maturity of data assets provides a baseline and guides organizations on improving the management of their data by applying best practices. You can test your data maturity with our free data management maturity self-assessment tool on Veracity. |
Data assurance is an integral part of digital trust, ensuring good quality data throughout its lifecycle. It ensures that data are trustworthy, accurate and fit for purpose. Data assurance is essential for building trust, making informed decisions and maximizing the value of data. |